The healthcare sector is at the forefront of the data revolution, generating massive amounts of data that have the potential to transform patient care. According to a study by Extract, the healthcare industry produces 11-14% of the world’s data. This includes electronic health records, clinical trials, and medical imaging data like CT scans and MRI scans.
With the rise of telemedicine and the increase in the number of digital health tools, the volume of data has overflowed, giving opportunities for data scientists in healthcare to extract actionable insights. The “quantified health” concept displays this shift, where data from wearable devices, connected gadgets, and other health-related technologies are seamlessly integrated into medical records. This integration is revolutionizing patient care, making it more personalized and data-driven than ever before.
The article discovers what data science covers in medicine and what is the role of data scientists in it. Let’s get into it!
How is data science used in healthcare?
Healthcare is a field like no other, where data isn’t just numbers on a screen—it’s the difference between life and death. Despite the vast amount of data generated in healthcare, from patient records to medical imaging, much of it remains overlooked. Data science comes into play by processing these arrays of information and directing them in innovative directions. See what can be done with data science in the health field:
Predictive Analytics for Early Disease Detection
Predictive analytics is a game-changer in healthcare, offering the ability to foresee potential health crises before they fully emerge. Data scientists can identify risk factors and predict disease outbreaks by analyzing patient data and using machine-learning models. According to insights from Deloitte, predictive analytics can provide significant value in healthcare by reducing hospital readmission rates and improving patient outcomes.
However, there are risks involved, including the potential for data privacy issues, algorithmic biases, and the need for healthcare providers to understand and trust the technology fully. The effectiveness of predictive models also depends heavily on the quality of the data and the robustness of the algorithms used.
One prominent example of work that data scientists have already done is the advanced technical tool MammaPrint™, which is known for breast cancer prognosis. This groundbreaking test, approved by the FDA (The United States Food and Drug Administration), analyzes a 70-gene signature to predict the risk of distant metastases. MammaPrint™ produces a score based on the weighted average of these genes, revolutionizing the way we approach breast cancer prognosis.
Drug Discovery
Traditional drug discovery is often slow and costly, but with data science in healthcare, researchers can rapidly analyze vast datasets to identify potential drug candidates. As stated in a report by Digital Science, data science enables researchers to mine existing data for new drug targets, predict drug responses, and optimize clinical trial designs.
This approach significantly accelerates the drug development pipeline, leading to faster delivery of new therapies to patients. Drawing on data science can help reduce the attrition rate in drug development by identifying early-stage failures, saving both time and resources.
Pattern recognition
Did you know that AI-powered software can identify heart murmurs with a level of accuracy that rivals or even surpasses that of expert cardiologists? This is just the beginning of what AI can achieve in clinical care.
Pattern recognition is one of the most powerful tools data science brings to healthcare. By analyzing complex healthcare data, AI and machine learning algorithms can detect subtle patterns that might go unnoticed by the human eye. Data scientists use advanced algorithms to uncover trends and correlations within patient data which might not be immediately apparent. This ability to detect patterns can lead to earlier and more accurate diagnoses.
Medical imaging
Data science plays a crucial role in advancing medical imaging technologies, which can help identify abnormalities in our organs. Insights from GoDataDrive say that AI and data science provide detailed information about the structure and functions of organs that were previously unattainable.
These technologies can analyze brain scans, identify minute changes in brain tissue, and predict the likelihood of disease progression with remarkable accuracy. The use of data science in brain imaging not only improves diagnostic capabilities but also enables more personalized treatment plans. By understanding the unique characteristics of each patient's brain, clinicians can tailor interventions, choose the best options for care,
Personalized Medicine and Targeted Treatments
Data science allows for the analysis of vast datasets, including genetic information, patient histories, and even lifestyle factors, to create highly individualized treatment plans. As highlighted in the BMC Medicine article "From Hype to Reality: Data Science Enabling Personalized Medicine," this approach is particularly beneficial in oncology, where treatments can be tailored to the specific genetic mutations present in a patient’s tumor, thereby improving treatment efficacy and reducing side effects.
This shift from a "one-size-fits-all" approach to a more personalized strategy in medicine has the potential to significantly improve patient outcomes and represents a fundamental shift in how healthcare is delivered.
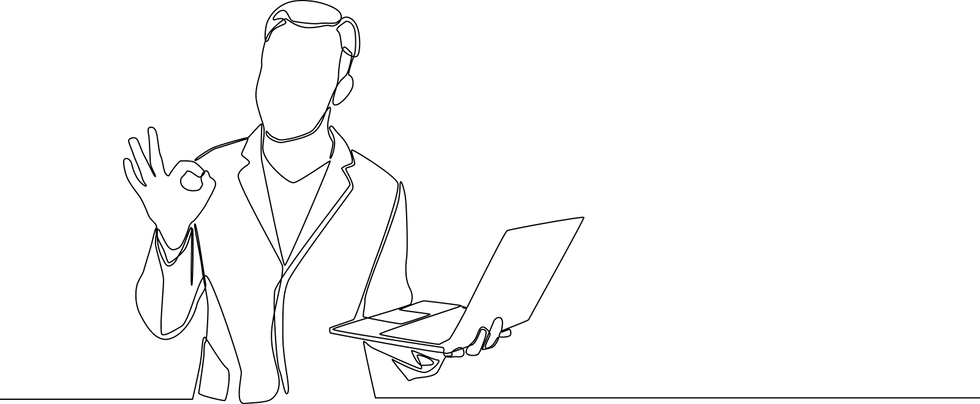
5 Successful Health-Tech Products
The integration of data science into healthcare has led to the development of many visionary health-tech products that are making a significant impact on patient care and medical research. Here are five standout examples:
Spatially Health
Spatially Health is using geospatial data analysis to address social determinants of health, particularly in underserved communities. By analyzing massive amounts of data related to demographics, location, and environmental factors, Spatially Health can identify areas where healthcare access is limited and target interventions to improve healthcare equity. This approach not only helps in reducing discrepancies in healthcare access but also supports better resource allocation, ensuring that those in need receive timely care.
We are successfully partnering with Spatially Health to find the best data science developers who will contribute to the healthcare industry from within, bringing their extensive expertise to drive further innovation and impact. Read our case study on Spatial Health to learn more about our approach to niche requests.
Tempus
Tempus is at the forefront of precision medicine, using genomic sequencing and data analytics to revolutionize cancer treatment. They operate with genetic data from patients to develop personalized cancer treatment plans that are tailored to specific mutations in a patient’s tumor. Tempus is not just changing the game; they’re rewriting the rules of cancer care.
Medisafe
Medisafe is turning medication management into a personalized experience that fits seamlessly into patients' lives. Using data science, Medisafe creates customized reminders and tracks adherence, ensuring that patients stick to their treatment plans. It’s not just about taking meds on time—it’s about transforming how patients engage with their health.
We at SD Solutions have been proud to partner with Medisafe for years, helping them build a dedicated offshore mobile team that’s been key to their success. Together, we’re ensuring that patients are supported every step of the way, leading to better health outcomes and quality of life.
Aidoc
Aidoc is revolutionizing radiology with AI-powered solutions that bring speed and accuracy to medical imaging. In a field where seconds can make all the difference, Aidoc’s technology helps radiologists quickly and accurately identify critical conditions. This means faster diagnoses, quicker treatments, and ultimately, more lives saved. Aidoc isn’t just supporting radiologists; it’s revolutionizing their work, making the diagnostic process more efficient and precise.
IBM Watson Health
IBM Watson Health is pushing the boundaries of what's possible in healthcare by using AI and machine learning to transform mountains of complex medical data into lifesaving insights. When traditional methods might struggle to find the right approach, Watson Health steps in with powerful, data-driven recommendations that empower clinicians to make smarter, faster decisions
What does a data scientist do in healthcare?
Data scientists in healthcare turn untapped data into actionable insights that revolutionize patient outcomes and streamline medical decisions. Those professionals need a specific set of skills to fulfill their responsibilities and make a meaningful impact in the health tech industry, including:
Data Collection & Interpretation:
Responsibilities: Gathering and interpreting healthcare data to support informed decision-making and improve patient outcomes.
Tech Stack: Python, SQL, R, ETL tools, Data Warehousing, Tableau, Power BI.
Data Management:
Responsibilities: Organizing and ensuring the accuracy of data from sources like electronic health records and clinical trials.
Tech Stack: SQL, Hadoop, Apache Spark, AWS/GCP/Azure, Database Management Systems (e.g., MySQL, PostgreSQL), Data Lakes.
Analysis & Modeling:
Responsibilities: Using advanced analytics and machine learning to uncover insights, predict outcomes, and optimize healthcare processes.
Tech Stack: Python (Pandas, NumPy, Scikit-learn), R, TensorFlow, PyTorch, MATLAB, Jupyter Notebooks, SAS.
Data Privacy:
Responsibilities: Protecting sensitive patient information with strong privacy measures and compliance with regulations.
Tech Stack: Encryption tools (e.g., SSL/TLS, AES), Compliance Management Software (e.g., HIPAA-compliant systems), Data Governance Tools, Firewalls, Cybersecurity Tools (e.g., SIEM systems).
Should a Data Scientist Have a Healthcare Experience Before Joining a HealthTech Company?
While healthcare experience isn’t a strict requirement for data scientists entering the health tech field, it’s a game-changer that can set you apart. Knowing the ins and outs of healthcare—like navigating complex privacy regulations and understanding the nuances of medical data—allows you to tackle industry-specific challenges with confidence. It also means you can hit the ground running, designing smarter models and interpreting data that directly improve patient outcomes. In a field where data can literally save lives, that’s a powerful advantage.
